Description
Explanations for model output decisions might be difficult, imprecise, or not possible to obtain.
Why is unexplainable output a concern for foundation models?
Foundation models are based on complex deep learning architectures, making explanations for their outputs difficult. Inaccessible training data could limit the types of explanations a model can provide. Without clear explanations for model output, it is difficult for users, model validators, and auditors to understand and trust the model. Wrong explanations might lead to over-trust.
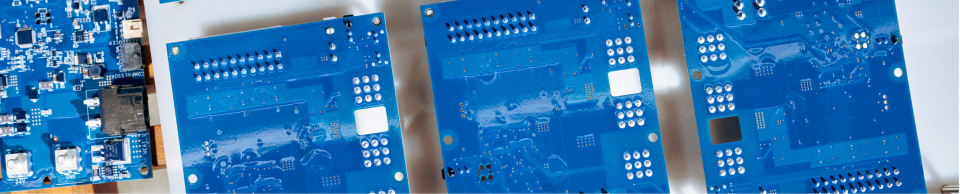
Unexplainable accuracy in race prediction
According to the source article, researchers analyzing multiple machine learning models using patient medical images were able to confirm the models’ ability to predict race with high accuracy from images. They were stumped as to what exactly is enabling the systems to consistently guess correctly. The researchers found that even factors like disease and physical build were not strong predictors of race—in other words, the algorithmic systems don’t seem to be using any particular aspect of the images to make their determinations.
Parent topic: AI risk atlas
We provide examples covered by the press to help explain many of the foundation models' risks. Many of these events covered by the press are either still evolving or have been resolved, and referencing them can help the reader understand the potential risks and work towards mitigations. Highlighting these examples are for illustrative purposes only.